Stripped out¶
Stéphanie Vilayphiou
Fabricademy 2024
Strip-p-ed Out¶
«Strip-p-ed Out» is a project researching patterns which can bypass human recognition of Computer Vision. What does a CCTV see? Does it really see humans or…? Can we fool its eye? Look at the camera which is looking at you looking at it. I eye I eye I eye I…
First ideas¶
Digital surveillance¶
The Media Design master I followed at Piet Zwart Institute (now called Experimental Publishing was focused on using new media/technologies to observe, criticize our world under socio-economical and political aspects, especially the impacts of digital technologies. During my graphic design practice, I was working a lot with Constant vzw, an organization for art & media, cyberfeminism in Brussels. I was working on making visible art projects close to the ones from my Masters degree. I would like to come back to this practice I left too long ago…
During my master at PZI, !Mediengruppe Bitnik, an artist duo, came to lead a workshop on CCTVs. I did not attend that workshop but I've heard about it from fellow students: they built a device which allowed them to walk around the city and see through the eyes of the CCTVs around them.

Extending that idea, I would like to make a device to not necessarily see what the cameras seen but rather feel where they are with a discrete device.
A garment or wearable accessory that would vibrate in the direction where a CCTV is detected. The walker can then decide to turn his·her face on the opposite side to avoid being seen. It is not a product that I want to sell, it’s a device meant to raise awareness of the massive presence of CCTVs. Therefore its circulation would be mostly through the web and artistic contexts.
The device is mostly thought for walking in the city, but it could actually also be used when going into private spaces (airbnb, hotels…) to detect if a CCTV is hidden somewhere…
- who
- general audience, probably more art-oriented
- what
- a device to detect CCTVs around
- when
- whenever
- where
- in the city, in private/rental spaces
- why
- to raise awareness of digital surveillance
- how
- a wearable and discrete device with multiple vibration motors which will vibrate in the direction of a CCTV

Ideas to extend the project¶
- Insecam feature
- On top of sensing CCTV, with the help of a GPS, the device could also tell the walker that the CCTV is available on Insecam, a website giving public access to unprotected CCTVs.
- Performance
- If several persons walking together are wearing the CCTV sensing device, maybe a form of choreography would emerge from it.
- Mapping
- If I have time, the detected CCTV position could be added to a map available publicly on the web.
- Camouflage
- If I have time, I would like to work on patterns for knitted garments/accessories to be invisible to human recognition systems (visuals, heat…)
Resources and tools
- Face detection browser with openCV by Gordan Savičić
- insecam: a website where one can watch unprotected CCTVs around the world
- autoknit: software to convert a 3D object into 3D knit instructions for industrial knitting machines, including Kniterate
- Bitnik, CCTV-A Trail of Images, Fanzine London, 2009
References around CCTVs¶
!Mediengruppe Bitnik¶
CCTV—A Trail of Images, 2008—2014
From 2008 to 2014, !Mediengruppe Bitnik conducted walks through the sceneries of invisible cities: equipped with self-built CCTV video signal receivers and video recording devices they organise dérives in search of hidden — and usually invisible — surveillance camera signals in public space. Surveillance becomes sousveillance: the self-built tools provide access to “surveillance from above” by capturing and displaying CCTV signals, thus making them visible and recordable.
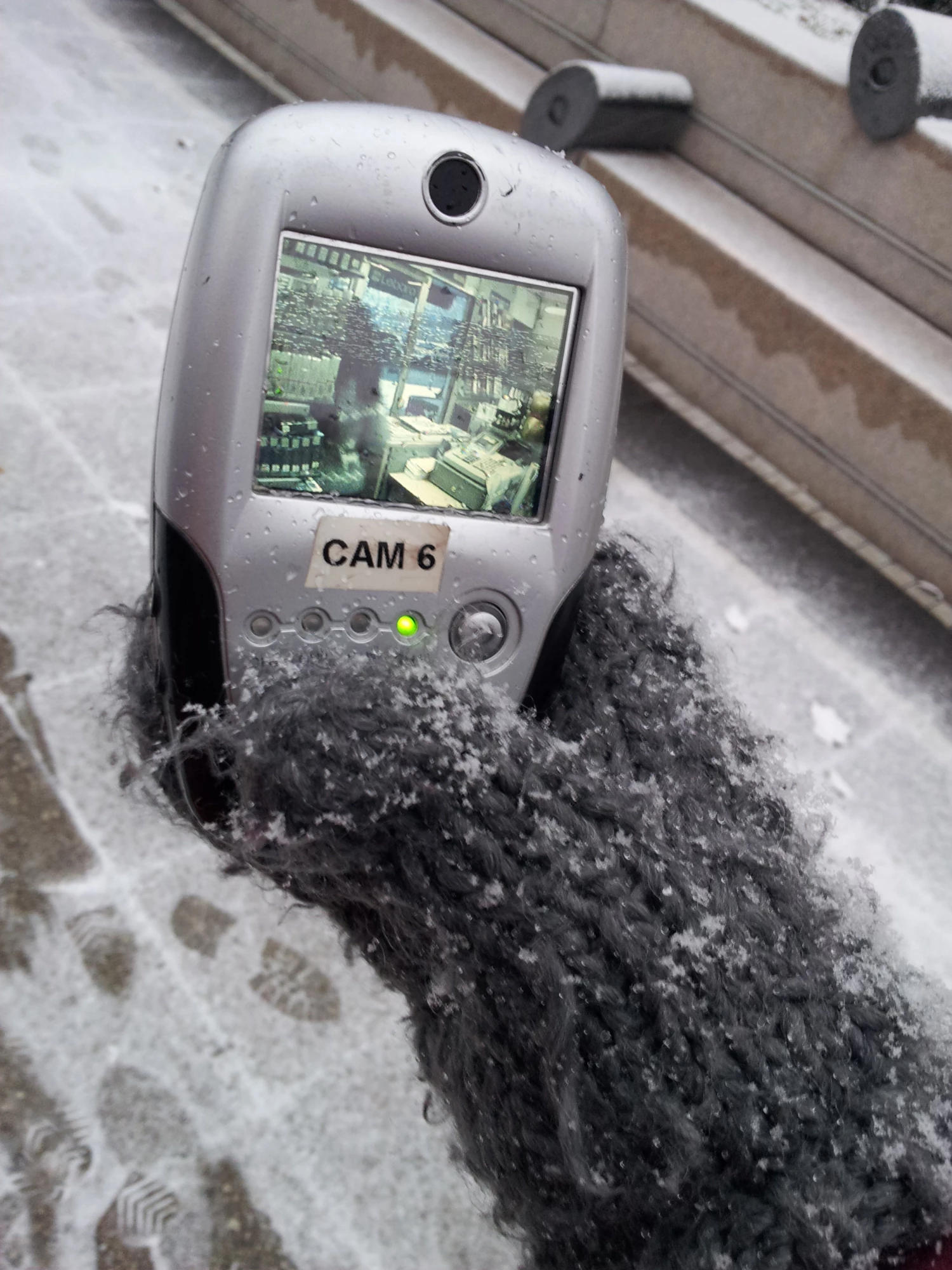
Militärstrasse 105, Hijacking Police Cameras, 2009
Zurich. In 2009, !Mediengruppe Bitnik hijacked the signals from two police surveillance cameras and rebroadcast them to the exhibition space.
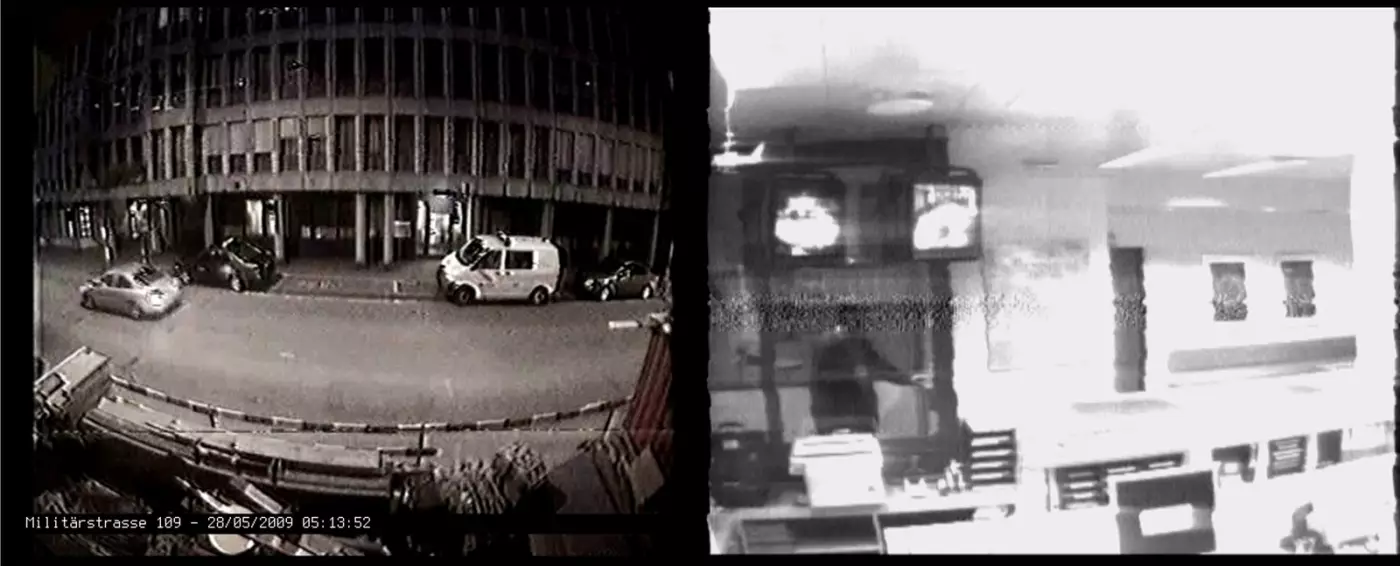
Surveillance Chess, Hijacking CCTV Cameras in London, 2012
London. 2012. On the brink of the Olympic Games. A tube station in one of the most surveilled public spaces in the world. !Mediengruppe Bitnik intercepts the signal of a surveillance camera: business people making their way to the Underground, a man in a suit looking for the right exit. From the left, a woman with a yellow suitcase walks into the frame of the surveillance camera. She opens her suitcase and activates a switch. This is the moment when Bitnik takes over. The surveillance image drops out, a chess board appears on the surveillance monitor and a voice from the loudspeakers says: ‘I control your surveillance camera now. I am the one with the yellow suitcase.’ The image jumps back to the woman with the yellow suitcase. Then the image switches to the chess board. ‘How about a game of chess?’, the voice asks. ‘You are white. I am black. Call me or text me to make your move. This is my number: 07582460851.’
SpY, Cameras, 2013¶
Spanish artist SpY made an art installation in Madrid covering a whole wall with fake CCTVs. I couldn't find back another art installation that I've heard about: in an art museum, a wall of CCTVs actually following the visitor when s·he walks across the room.

"Laboratoire de surveillance - football & supporterisme", Théo Hennequin, 2023¶
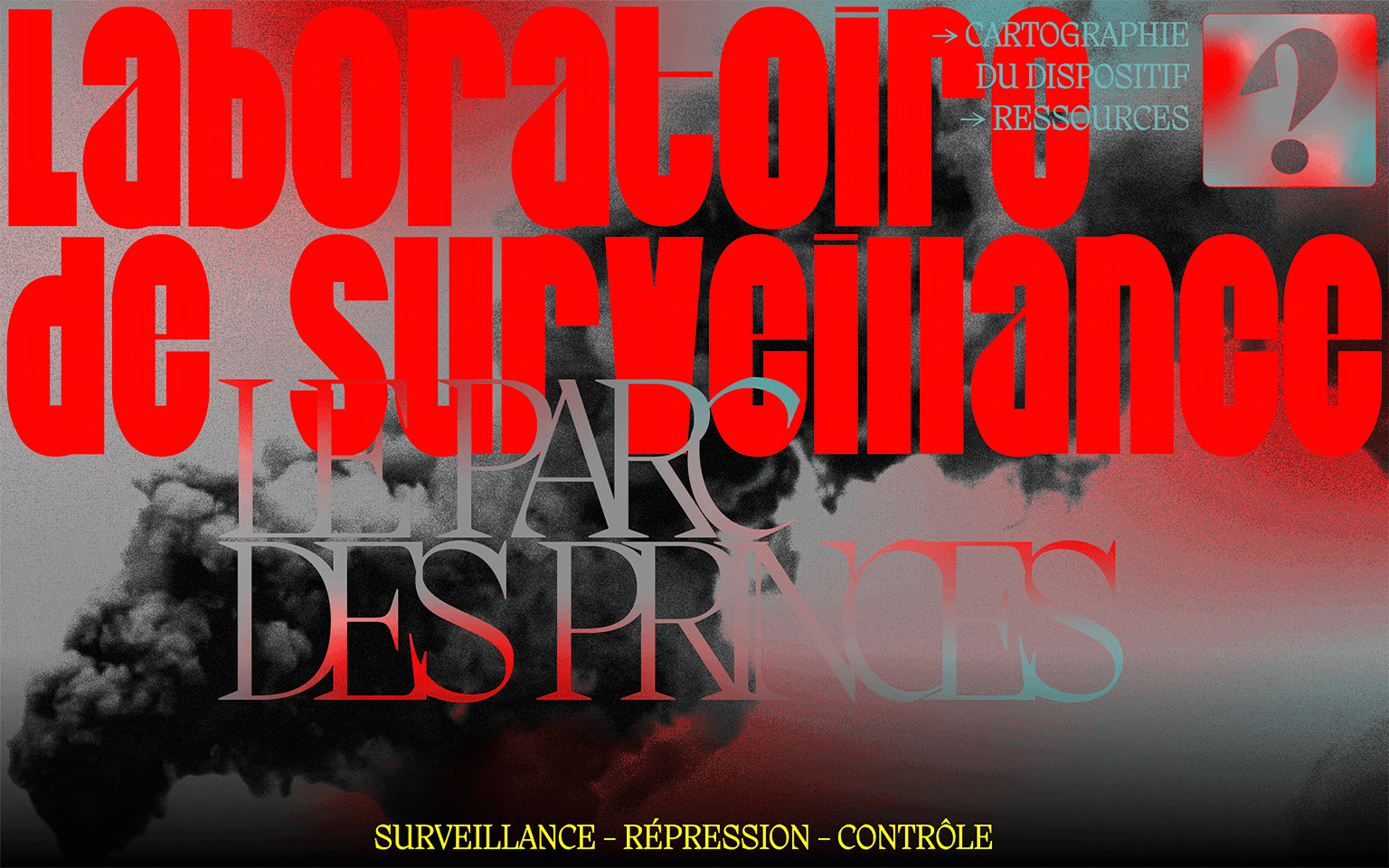
The book "Laboratoire de surveillance - football & supporterisme" explores the mechanisms of surveillance, repression and control to which football supporters, Ultras, fervent supporters and protesters are subjected. These measures, which have been tested in stadiums, include policing techniques, facial recognition, group disbanding, administrative bans, proactive judicial laws, arbitrary convictions without trial and police violence. Football stadiums are being used as a surveillance laboratory to experiment with these devices, with repercussions for activism and demonstrations. The case analysis presented in the book focuses on the Parc des Princes in Paris, but the devices are similar in many other stadiums. The visual and textual treatment of the case study is the result of research carried out by a graphic designer. The book highlights the liberticidal risks of these devices in a context of growing social tensions, marked by increasing inequality and discrimination.
References around camouflage¶
Adam Harvey¶
Make-up and hairstyle based on asymmetry to bypass facial recognition. According to the article «‘Dazzle’ makeup won’t trick facial recognition. Here’s what experts say will», this technique no longer works since 2020. But Harvey stipulates that the same reverse-engineering technique can be applied to find new shapes to bypass current systems. Although this solution makes you invisible for machines, it makes you over-visible for humans.
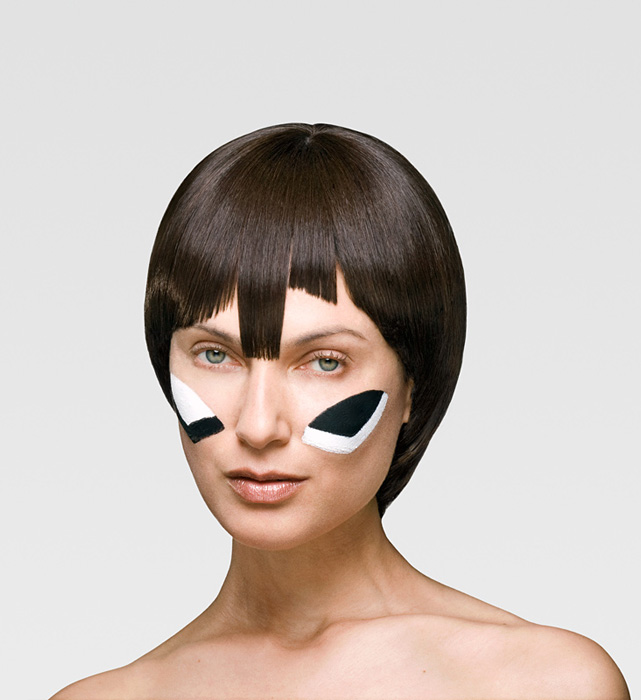
Later on, Harvey worked a textile pattern which could be recognized as faces, thus hiding the wearer's face from the image recognition software. This solution is more efficient than the latter as it makes you less over-visible for humans.
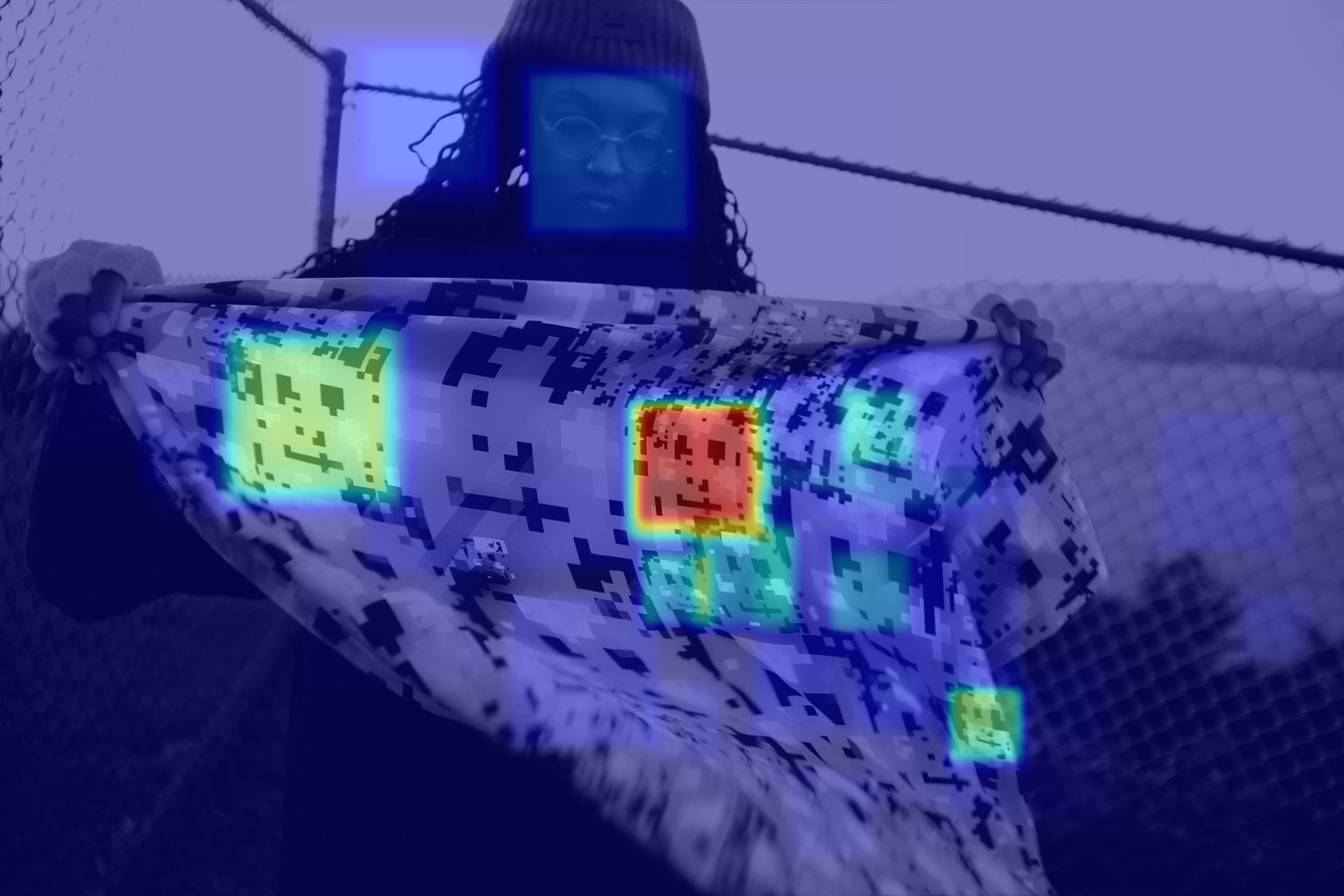
A Clutch that emits a super strong light when a camera flash is detected in order to overexpose the photo.

Garments made of silver-plated fabric to avoid thermal recognition from drones.

Faking Animals¶
Cap_able is an Italian design studio working on high-tech products that «open the debate on issues of our present that will shape our future». I find these designs really interesting as they are eccentric but they don't look too weird either, therefore you are not overvisible by humans.

Car prototypes camo¶

Addie Wagenknecht, Anonymity, 2007¶
Artist Addie Wagenknecht has distributed "anonymity" glasses to people in a city center: these glasses are just simple sunglasses in a rectangular shape. This type of performance is really inspiring for my project here. I really like the simple but yet super efficient strategy.
References around translating digital into tangible¶
Gordan Savičić, Constraint City, The pain of everyday life, 2007¶
Austrian artist Gordan Savičić built a strapping vest which would tighten when encountering encrypted wifi signals. The stronger the signal, the tighter the vest.

Quick & dirty prototypes¶
Micro:bit¶
The Micro:bit micro-controllers can communicate via radio frequencies between each other. I tried capturing frequencies from FM radio or talkie-walkie frequencies by looping through groups but nothing seemed to came up. Here is the Python code anyways:
Code
from microbit import *
import radio
# Code in a 'while True:' loop repeats forever
while True:
# A checkmark icon when booting the program
display.show(Image.YES)
sleep(1000)
# Turning radio frequencies on
radio.on()
# Looping from 0 to 255
for i in range(0,255):
# Changing group from 0 to 255
radio.config(group=i, power=0)
# Receiving a signal
message = radio.receive()
# If a message is received,
# a skull icon should appear on the LEDs
if message:
display.show(Image.SKULL)
sleep(400)
sleep(1000)
After this quick try, I was a bit dubious about the CCTV detection cape. The system used by !Mediengruppe Bitnik was a 2.4GHz radio frequency receiver. It is quite large (size of a hand), and I was dubious about its capacity to locate the camera in the space.
Therefore I contacted the French artist Benjamin Gaulon who made a project in 2008 revealing CCTVs images in the street on small screens right underneath their location. He answered me quickly that:
- CCTVs are closed-circuit systems impossible to dive in
- Most surveillance cameras nowadays don’t work with radio frequencies anymore but with IP systems through private wireless networks.
Based on those observations, I decided to abandon the CCTV detection wearable device to focus on digital camouflage.
Instagram filters¶
In order to know what patterns I should make for digital camouflage, I first need to understand what is recognized and what's not. As quick and dirty tests, I started with what I had available close to me: Instagram filters. I tried the filter by putting my laptop screen in front of the camera so that I could easily try different types of images. I made tests on pictures from Adam Harvey’s projects and from Rachele Didero’s Capable project.
Filter: My Tiny Army¶
I used the filter «My Tiny Army» as some filters work only for one face/person at the same time. The filter could detect people on the Harvey and Didero’s work. But it worked also on animals and flowers, therefore we can conclude that the filter just extracts a foreground subject from the background. These results are thus non-relevant. It output funny patterns though.
















Filter: Juliet¶
I thus tried another filter for multiple faces. It worked on humans and not animals, but only with facing people and not from profile.





OpenCV¶
I just added an argument to execute the script while specifying a filename. You can execute the code like this where test-opencv.py contains the code below python test-opencv.py "myImage.jpg"
.
Code from https://www.datacamp.com/tutorial/face-detection-python-opencv
# Importing libraries
import cv2
import sys
import matplotlib.pyplot as plt
# Image filepath is specified when executing the script from the command line
imagePath = '%s' % sys.argv[1]
# Reads the image
img = cv2.imread(imagePath)
img.shape
# Converts to grayscale for faster process
gray_image = cv2.cvtColor(img, cv2.COLOR_BGR2GRAY)
# Loading face classifier
face_classifier = cv2.CascadeClassifier(
cv2.data.haarcascades + "haarcascade_frontalface_default.xml"
)
face = face_classifier.detectMultiScale(
gray_image, scaleFactor=1.1, minNeighbors=5, minSize=(40, 40)
)
for (x, y, w, h) in face:
cv2.rectangle(img, (x, y), (x + w, y + h), (0, 255, 0), 4)
img_rgb = cv2.cvtColor(img, cv2.COLOR_BGR2RGB)
plt.figure(figsize=(20,10))
plt.imshow(img_rgb)
plt.axis('off')
plt.show() # This line was missing from the original code, without it, it won't display the image.
Human body detection with OpenCV¶
Code from https://thedatafrog.com/en/articles/human-detection-video/ taking a webcam as input. It works when the human is not too close from the camera. I am not documenting this code, I just copy/paste it like the original.


Code
# Code from <https://thedatafrog.com/en/articles/human-detection-video/>
# import the necessary packages
import numpy as np
import cv2
# import the necessary packages
import numpy as np
import cv2
# initialize the HOG descriptor/person detector
hog = cv2.HOGDescriptor()
hog.setSVMDetector(cv2.HOGDescriptor_getDefaultPeopleDetector())
cv2.startWindowThread()
# open webcam video stream
cap = cv2.VideoCapture(0)
# the output will be written to output.avi
out = cv2.VideoWriter(
'output.avi',
cv2.VideoWriter_fourcc(*'MJPG'),
15.,
(640,480))
while(True):
# Capture frame-by-frame
ret, frame = cap.read()
# resizing for faster detection
frame = cv2.resize(frame, (640, 480))
# using a greyscale picture, also for faster detection
gray = cv2.cvtColor(frame, cv2.COLOR_RGB2GRAY)
# detect people in the image
# returns the bounding boxes for the detected objects
boxes, weights = hog.detectMultiScale(frame, winStride=(8,8) )
boxes = np.array([[x, y, x + w, y + h] for (x, y, w, h) in boxes])
for (xA, yA, xB, yB) in boxes:
# display the detected boxes in the colour picture
cv2.rectangle(frame, (xA, yA), (xB, yB),
(0, 255, 0), 2)
# Write the output video
out.write(frame.astype('uint8'))
# Display the resulting frame
cv2.imshow('frame',frame)
if cv2.waitKey(1) & 0xFF == ord('q'):
break
# When everything done, release the capture
cap.release()
# and release the output
out.release()
# finally, close the window
cv2.destroyAllWindows()
cv2.waitKey(1)
I mixed the former two codes to be able to detect human bodies but on still images. In green are detected faces and in yellow are detected bodies. I first started with random open source pictures from Google Images, then I thought it would be nice to use pictures of women in tech rather than random content.

Given the results, I wanted to check how much of the body is needed for the software to recognize a human. So I cropped a positive result to test that.







Tests on Capable.design pictures¶





Code
#! python
import cv2
import numpy as np
import sys, os
import pathlib
# initialize the HOG descriptor/person detector
hog = cv2.HOGDescriptor()
hog.setSVMDetector(cv2.HOGDescriptor_getDefaultPeopleDetector())
# loads face classifier tool
face_classifier = cv2.CascadeClassifier(
cv2.data.haarcascades + "haarcascade_frontalface_default.xml"
)
# get input and output paths from the command line
# ./human-detection.py "../input/" "../output/"
inputPath_str = sys.argv[1]
inputPath = pathlib.Path(inputPath_str)
# Sets variables according if the input is a file or a folder
if inputPath.is_dir():
folder = True
images = os.listdir(r'%s' % inputPath)
else:
folder = False
outputPath = '%s' % sys.argv[2]
# Main function
def analyseImage(image, filename):
img = cv2.imread(image)
img.shape
# converts image to grayscale image for faster processing
gray_image = cv2.cvtColor(img, cv2.COLOR_BGR2GRAY)
# detect people in the image
boxes, weights = hog.detectMultiScale(gray_image, winStride=(8,8) )
# returns the bounding boxes for the detected objects
boxes = np.array([[x, y, x + w, y + h] for (x, y, w, h) in boxes])
for (xA, yA, xB, yB) in boxes:
# display the detected boxes in the colour picture
cv2.rectangle(img, (xA, yA), (xB, yB), (0, 255, 255), 2)
# detects faces in the image
face = face_classifier.detectMultiScale(
gray_image, scaleFactor=1.1, minNeighbors=5, minSize=(40, 40)
)
for (x, y, w, h) in face:
# display the detected boxes in the colour picture
cv2.rectangle(img, (x, y), (x + w, y + h), (0, 255, 0), 2)
img_rgb = cv2.cvtColor(img, cv2.COLOR_BGR2RGB)
# Saves output images in outputPath folder
if folder and outputPath:
print(filename)
cv2.imwrite(filename, img)
# Shows output in a new window
else:
import matplotlib.pyplot as plt
plt.figure(figsize=(20,10))
plt.imshow(img_rgb)
plt.axis('off')
plt.show()
# If the input path is a folder
# applies script to all images
if folder:
for img in images:
analyseImage(inputPath_str + img, outputPath + img)
# Otherwise just treat one image and shows it
else:
analyseImage(inputPath_str, outputPath + inputPath_str)
To launch the script for several files at the same time, I used a simple bash loop:
for FILE in ../input-bodies/*; do python human-detection.py $FILE; done
References¶
- [ ] https://www.mdpi.com/1424-8220/20/2/342
- [x] https://www.vice.com/en/article/aen5pz/countersurveillance-textiles-trick-computer-vision-software
- [ ] https://github.com/sourabhvora/HyperFace-with-SqueezeNet
- [ ] https://pypi.org/project/knit-script
- [ ] OpenCV Cascade classifiers: https://docs.opencv.org/3.4/db/d28/tutorial_cascade_classifier.html
- PimEyes is a Face Search Engine Reverse Image Search. You can upload one or several photos of a face, and it will search the web for pictures of the same person.
Kniterate¶
In parallel to computer vision tests, I made some samples on the Kniterate, a semi-industrial knitting machine as I plan to knit my digital camouflages with this machine. See this page to look at those experiments.
Kniterate¶
In parallel to computer vision tests, I made some samples on the Kniterate, a semi-industrial knitting machine as I plan to knit my digital camouflages with this machine.
Yarns¶
Kniterate is a 7 gauge machine (7 needles per inch) and welcomes yarns from Nm6 to Nm8.
The most difficult thing to tackle with the Kniterate are yarns which will go flawlessly in the machine, either for the waste yarn (a yarn you don’t want to put too much money into) and the knit yarn. For the waste yarn, we used PLA yarns made out of corn from Noosa Fibers. After removing the waste yarn, we could potentially send them back to the company for recycling.
Birds-eye jacquard, inverted colors (full-needle rib)¶




Birds-eye jacquard, 3 colours, default options¶



Birds-eye jacquard, 3 colours, Full-needle rib¶
Instagram post embedding not available.Watch it on Instagram
dithering tests¶
In the idea of making countershadows, I tried different dithering software/settings to make a radial gradient with the Kniterate.










Prototypes¶

More tests on «in the wild» images¶
I ran more tests on different categories of images to understand more how the machine recognizes humans.
Faces¶


Environment¶


Human or biped recognition?¶




Changing the silhouette drastically might work but not all the time.¶



Patterns¶






Feedback from midterm presentation with Ricardo O'Nascimento¶
Love how you showed us your research today - makes it very clear, and very relatable, yet to the point.
This would be a great part in your video - its super communicative. What do you expect to be the final outcome? if it is a full garment - what are your first suspicious of which elements will work best? do you have already preliminary outcomes - hints of what you will use to make the design choices? I am a big fan of your concept :) if you are passing by amsterdam any time soon - i have a number of colleagues that i think you will have great conversations with about both privacy, survaillance, encrypting messages into clothing, etc etc :D"
The project concept is great, yet, the presentation should be more organized and formal.
The project is very challenging, yet most of the work it is done. Congratulations again on the studies with camera and facial recognition. Deciding what to knit and why is your next focus, I like the suggestions of photochromic inks and the idea of embedding data visualization. Stripes generated from surveillance data is a valid point. I like the kimono ideas!
I came across this recently...I think you might find it interesting. Dazzle Camoflage https://www.slashgear.com/947328/what-is-dazzle-camouflage/. Great idea to mark up images to see its impact on Image Recognition algorithm.
Small-scale prototypes¶
I started prototyping in small scale with my daughter’s Barbie dolls (I’ve never bought any and they don’t like Barbies anyways, so it’s perfect, haha…). I made this small very simple rectangular structure to make a kimono-like vest which is easy to construct and to put on.




video



Next steps¶
- Use datasets around camera surveillance to generate stripes which have meaning. Colors, thicknesses can be used to translate data. Where to find free datasets:https://careerfoundry.com/en/blog/data-analytics/where-to-find-free-datasets/
- Collective CCTV maps: https://sunders.uber.space/ or https://osmcamera.dihe.de/
-
Top CCTVs:
-
Cryptography researcher Seda Gürses claims that the more one is hiding from digital surveillance (talking about web surveillance in this case), the more that person is actually identified. Adam Harvey also claims that the more we fight surveillance systems, the stronger it gets. So instead of hiding, an attack could actually to pollute captured data with false data, either wrong or too many. How to achieve that within a garment?
- pick up second-hand/torn clothes from thrift shops for people in the exhibition to draw onto an anti-recognition pattern and take away their cloth → use big brushes with natural inks
Final proposals¶
Dolls¶
For the exhibition in May, I would like to exhibit the small scale prototypes I've made. Besides, I will invite the audience to make their own prototype and test it with a live OpenCV. They can later bring their prototype home and upscale their pattern on a real garment. To ease the prototyping, I made a lasercut template to assemble the garment with tabs and notches rather than sewing.
Hand-drawn tests with Posca and textile markers:




Tests with OpenCV of different patterns:












Vest 1: Readymade¶
Some patterns might already be available for a good camouflage. You must pay attention to the orientation though, it looks like horizontal patterns work best. Here are some examples with fabric found at Green Fabric:






I used one the pink and yellow striped fabric to make a very fast test of a scale 1 vest. It is an assembly of rectangles, it could use better shaping, but it's good for a prototype.



Vest 2: Scribbling¶
I used the "Hatches" effect for objects in Inkscape to make scribbling on the panels. It was quite useful to manage the thicknesses and orientaion of the lines.

It's the first time that I made a big and finished object with the Kniterate! It was quite exciting. I had a lot of holes that I had to repair because the yarns were too fine/fragile.
Source files





Vest 3: Dataviz¶
The thicknesses of the stripes of this vest are translations of the amound of CCTVs in 5 European countries.
Data is from 2023, retrieved on this website: https://www.comparitech.com/vpn-privacy/the-worlds-most-surveilled-cities/. It is a top 150 of the most surveilled cities in the world, there are only 5 European ones in this list! Those are the ones I kept for the dataviz.





The captions of the dataviz have been lasercut:

Source files
Final vest:



Video¶
Video available at https://videos.domainepublic.net/w/56Epknx9rmjKCh6d9QCX1f
Draft storyboard¶
Voiceover: text-to-speech
Screenshots¶









































































Reading notes¶
Contributors of Wikipedia, «Camouflage», retrieved on March 2024¶
The majority of camouflage methods aim for crypsis, often through a general resemblance to the background, high contrast disruptive coloration, eliminating shadow, and countershading.
Steven Porter, «Can this clothing defeat face recognition software? Tech-savvy artists experiment», The Christian Science Monitor, 2017¶
«That’s a problem,» Dr. Sellinger and Dr. Hartzog wrote. «The government should not use people’s faces as a way of tagging them with life-altering labels. The technology isn’t even accurate. Faception’s own estimate for certain traits is a 20 error rate. Even if those optimistic numbers hold, that means that for every 100 people, the best-case scenario is that 20 get wrongly branded as a terrorist.»
[…] «I think camouflage is often misunderstood as a Harry Potter invisibly cloak, when camouflage actually is about optimizing the way you appear and reducing visibility.»
Kate Mothes, «Trick Facial Recognition Software into Thinking You’re a Zebra or Giraffe with These Pyschedelic Garments», Colossal, 2023¶
«Choosing what to wear is the first act of communication we perform every day. (It’s) a choice that can be the vehicles of our values, » says co-founder and CEO Rachel Didero. Likening the commodification of data to that of oil and its ability to be sold and traded by corporations for enormous sums—often without our knowledge—Didero describes mission of Cap_able as «opening the discussion on the importance of protecting against the misuse of biometric recognition cameras.» When a person dons a sweater, dress, or trousers woven with an adversial image, their face is no longer detectable, and it tricks the software into categorizing them as an animal rather than a human.
Adam Harvey, «On computer vision», * UMBAU: Political Bodies*, 2021¶
Photography has become a nineteenth-century way of looking at a twenty-first century world. In its place emerged a new optical regime: computer vision.
Computer vision, unlike photography, does not mirror reality but instead interprets and misinterprets it, overlaying statistical assumptions of meaning. There is no truth in the output of computer vision algorithms, only statistical probabilities clipped into Boolean states masquerading as truthy outcomes with meaning added in post-production.
Face detection algorithms, for example, do not actually detect faces, though they claim to. Face detection merely detects face-like regions, assigning each with a confident score.
Algorithms are rule sets, and these rules are limited by the perceptual capacities of sensing technologies. This creates «perceptual topologies» that reflect how technology can or cannot see the world. In the first widely used face detection algorithm, developed in 2001 by Viola and Jones, the definition of a face relied on the available imagery of the time for training data. This comprised blurry, low resolution, grayscale CCTV imagery. The Viola-Jones face detection algorithm mirrored back the perceptual biases of low-resolution CCTV systems from the early 2000’s by encoding a blurry, noisy, grayscale definition of the human face. Understanding this perceptual topology can also help discover perceptual vulnerabilities. In my research for CV Dazzle (2010) and HyperFace (2016) I showed that the Viola-Jones Haar Cascade algorithm is vulnerable to presentation attacks using low-cost makeup and hair hacks that obscure the expected low resolution face features, primarily the nose-bridge area. By simply inverting the blocky features of their Haar Cascade algorithm with long hair or bold makeup patterns, faces could effectively disappear from security systems. Another vulnerability of the Haar Cascade algorithm is its reliance on open-source face detection profiles, which can be reverse-engineered to produce the most face-like face. In 2016, I exploited this vulnerability for the HyperFace project to fool (now outdated) face detection systems into thinking dozens of human faces existed in a pink, pixellated graphic on a fashion accessory.
In Paglen’s ImageNet Roulette he excavates the flawed taxonomies that persisted in the WordNet labeling system that was used to label ImageNet, then purposefully trained a flawed image classification algorithm to demonstrate the dangers of racist and misogynistic classification structures.
Becoming training data is political, especially when that data is biometric. But resistance to militarized face recognition and citywide mass surveillance can only happen at a collective level. At a personal level, the dynamics and attacks that were once possible to defeat the Viola-Jones Haar Cascade algorithm are no longer relevant. Neural networks are anti-fragile. Attacking makes them stronger. So-called adversarial attacks are rarely adversarial in nature. Most often they are used to fortify a neural network. In the new optical regime of computer vision every image is a weight, every face is a bias, and every body is a commodity in a global information supply chain.
Adam Harvey, «Origins and endpoints of image training datasets created “in the wild”», 2020¶
The new logic is not better algorithms; it is better data, and more data.
In 2016, a researcher at Duke University in North Carolina created a dataset of student images called Duke MTMC, or multi-targeted multi-camera. The Duke MTMC dataset contains over 14 hours of synchronized surveillance video from 8 cameras at 1080p and 60FPS, with over 2 million frames of 2,000 students walking to and from classes. The 8 surveillance cameras deployed on campus were specifically setup to capture students «during periods between lectures, when pedestrian traffic is heavy». The dataset became widely popular and over 100 publicly available research papers were discovered that used the dataset. These papers were analyzed according to methodology described earlier to understand endpoints: who is using the dataset, and how it is being used. The results show that the Duke MTMC dataset spread far beyond its origins and intentions in academic research projects at Duke University. Since its publication in 2016, more than twice as many research citations originated in China as in the United States. Among these citations were papers linked to the Chinese military and several companies known to provide Chinese authorities with the oppressive surveillance technology used to monitor millions of Uighur Muslims.
From one perspective, «in the wild» is an ideal characteristic for training data because it can provide a closer match to an unknown deployment environment. Theoretically, this can improve real-world performance by reducing disparity and bias. In reality, data collected from sources «in the wild» inherit new problems including the systemic inequalities within society and are never «natural» or «wild». Representing datasets as unconstrained or «wild» simplifies complexities in the real world where nothing is free from bias. Further, collecting data without consent forces people to unknowingly participate in experiments which may violate human rights.
It is advisable to stop using Creative Commons for all images containing people.
Adam Harvey, «What is a Face?», 2021¶
Computer vision requires strict definitions. Face detection algorithms define faces with exactness, although each algorithm may define these parameters in different ways. For example, in 2001, Paul Viola and Michael Jones introduced the first widely-used face detection algorithm that defined a frontal face within a square region using a 24 × 24 pixel grayscale definition. The next widely used face detection algorithm, based on Dalal and Trigg’s Histogram of Oriented Gradients (HoG) algorithm, was later implemented in dlib and looked for faces at 80 × 80 opixels in grayscale. Though in both cases images could be upscaled or downscaled, neither performed well at resolutions below 40 × 40 pixels. Recently, convolutional neural network research has redefined the technical meaning of face. Algorithms can now reliably detect faces smaller than 20 pixels in height, while new face recognition datasets, such as TinyFace, aim to develop low-resolution face recognition algorithm that can recognize an individual at around 20 × 16 pixels.
As an image resolution decreases so too does the dimensionality of identity.
Quentin Noirfalisse, «Courtrai, reconnaissance faciale dans le viseur ? S’équiper pour surveiller. Épisode 4», in «Hypersurveillance policière», Médor web, 23/12/2021¶
Briefcam possède un outil assez pratique pour des policiers débordés : le Vidéo Synopsis. Il peut vous résumer des heures de vidéos en quelques minutes, en agglomérant des « objets » (individus, véhicules, par exemples) qui sont passés à différents moments sous l’oeil des caméras. En 2019, Vincent Van Quickenborne en faisait une présentation on ne peut plus enthousiaste aux médias. « Le logiciel va aller rechercher tous les gens qui portent un sac à dos, des véhicules de couleur rouge ou qui contiennent un chien. La direction, la taille et la vitesse de l’objet peuvent être évalués. »
Là est tout l’enjeu posé par l’arrivée d’une solution comme Briefcam dans l’arsenal policier en Belgique. La reconnaissance faciale est interdite par la loi belge. Nous sommes un des deux pays européens, avec l’Espagne, à ne pas le permettre. Pourtant, Briefcam, de l’aveu même de la police courtraisienne à l’époque, et selon ses propres plaquettes publicitaires, dispose d’une telle fonction.
La société RTS, qui détient une licence d’importateur pour Briefcam et l’a installé à Courtrai, a dû, à l’époque, désactiver les droits d’utilisateur pour la reconnaissance faciale. L’option est automatiquement disponible. RTS se justifie : leurs fournisseurs « supposent que tout le monde veut faire usage de la reconnaissance faciale ».
Un seul exemple : comme le montre le projet Gendershades du Massachussets Institute of Technology, si on est une femme ou une personne à la peau foncée, on a plus de chances d’être victime d’une erreur d’identification qu’un bon vieux mâle blanc.
Olivier Bailly, «Mais où est Johan ? BNG, la base non-gérée (4/5)», in «Hypersurveillance policière», 28/04/2021¶
Johan s’est vu refuser son accès à une activité professionnelle pour ça (extrait de la réponse de l’Autorité nationale de Sécurité) :
Sophie
Elle est militante écolo, de gauche, tantôt radicale, tantôt consensuelle. C’est une chercheuse aussi. En 2019, elle est engagée à l’Agence fédérale de contrôle nucléaire (AFCN).
Elle a à peine signé son contrat que la voilà licenciée. Elle n’a pas obtenu son habilitation de sécurité. « J’aurais été virée pour mon passé militant, j’aurais trouvé cela dégueulasse mais bon, j’aurais compris. Mais ce n’est pas cela que j’ai trouvé dans mon dossier ».
Car Sophie a été en recours. Et quand elle a pu compulser les pages la concernant, elle a d’abord trouvé ce à quoi elle s’attendait : la participation à des manifestations et événements, certains où elle était présente, d’autres pas. On y signale trois arrestations administratives lors de manifestations. Sophie ne conteste pas.
Mais aucune poursuite judiciaire n’a été menée et, dans son argumentaire, son avocat précise qu’« un grand nombre de personnes ont été arrêtées en même temps que ma cliente ».
Accessoirement, les manifestations datent de 2014. Elles auraient du être archivées en 2017.
Mais surtout, Sophie a appris son appartenance à un groupe extrémiste qui véhicule son lot de haine et que Sophie n’a jamais fréquenté.
Olivier Bailly, «Le grand quizz de la BNG», in «Hypersurveillance policière», 04/05/2021¶
En 2017, un événement étonnant (Tomorrowland) sera passé au crible de la BNG. 50 000 personnes. C’était du côté de la Côte.
Le but de ce screening massif était de contrôler si les festivaliers étaient connus de la police pour certains faits commis dans une période déterminée. C’est au final 29 186 identités de visiteurs (et 21 433 identités de collaborateurs) qui ont été analysées ! 10 % étaient connues de la BNG, soit 2 077 visiteurs et 1 912 collaborateurs.
Si on comptait 31 000 enquêtes encodées fin 2006, il y en avait 270 000 en 2019, soit neuf fois plus d’enquêtes encodées. De quoi inventer le néologisme « factobésité » ! Et encore, c’était en 2019. Depuis lors, le coronavirus et sa cohorte de PV sanitaires sont venus s’ajouter au mégalodon.
Nous avons donc appris que la BNG a fonctionné sans loi claire et précise pendant 12 ans, que plus de trois millions de suspects y étaient recensés en 2019 et que parmi eux, des mineurs de 14 ans pouvaient se retrouver encodés sans autorisation de magistrat de la Jeunesse. Prêts pour la suite ?
Lors des auditions pour confectionner la loi de 2014, quand la BNG ne pesait alors « que » 1,7 millions de personnes enregistrées, les mineurs de 14 à 18 ans représentaient environ 15 % du nombre de personnes identifiées. Les moins de quatorze ans, 1,6 %.
« 52 % des dossiers traités en 2019 se sont soldés par un effacement complet ou partiel des enregistrements effectués par la police dans la BNG ».
52 % ! Conclure que la moitié des infos dans la BNG est fausse, ce serait un raccourci excessif. Les personnes qui ont demandé une vérification suspectaient peut-être que leur dossier contenait des erreurs. Cependant, la conclusion du COC ne laissait pas beaucoup de doutes : « (…) la BNG contient encore de nombreuses inexactitudes et/ou erreurs. »
Sans archivage, on commence à être vraiment serrés dans la BNG. Surtout avec la croissance exponentielle de PV liés au coronavirus. C’est d’autant plus énorme que début mars 2021, le gouvernement a envisagé une mesure toute particulière pour les personnes de retour de voyage à l’étranger.
Les personnes recevraient un SMS les enjoignant de faire un test Covid ou une mise en quarantaine. Si elles ne s’exécutent pas, direction une base de données policières !
«Pour l’interdiction de la reconnaissance faciale à Bruxelles», petition, March 2023¶
La reconnaissance faciale menace nos libertés
L’usage de cette technologie dans nos rues nous rendrait identifiables et surveillé·es en permanence. Cela revient à donner aux autorités le pouvoir d’identifier l’intégralité de sa population dans l’espace public, ce qui constitue une atteinte à la vie privée et au droit à l’anonymat des citoyen·nes. La surveillance musèle la liberté d’expression et limite les possibilités de se rassembler, par exemple lors de manifestations. La reconnaissance faciale impactera surtout les groupes sociaux particulièrement affectés et marginalisés : personnes migrantes, communauté LGBTQI+, minorités raciales, personnes sans-abri, etc.
- risques quant au stockage des données :
les risques de piratages informatiques visant ces données biométriques très sensibles sont importants et l’actualité belge a, de nombreuses fois, montré que les données récoltées par les autorités publiques n’étaient pas à l’abri de ces piratages ;- risques d'erreurs et de discriminations accrues :
les études montrent que cette technologie reproduit les discriminations sexistes ou racistes induites par les conceptions sociales dominantes et des institutions qui les vendent et qui les utilisent ;- risques de normalisation et de glissement vers la surveillance de masse :
le déploiement des technologies de surveillance avance à coups de projets pilotes qui précèdent les cadres légaux, puis sont ensuite régularisés, souvent sans débat démocratique.
Examen de la pétition contre l’usage de la reconnaissance faciale en Région de Bruxelles-Capitale, 13 juin 2023¶
Que dit la loi ?
En Belgique, aucune loi ne réglemente l’usage de la technologie de reconnaissance faciale. Comme je viens de le dire, il s’agit pourtant de données biométriques, des données uniques, propres à chacun – notre visage, nos empreintes digitales, nos iris, par exemple. C’est ce qui rend ces données si intéressantes pour l’identification des personnes, mais également si dangereuses lorsqu’elles sont utilisées à mauvais escient. Le traitement des données biométriques a un impact majeur sur notre vie privée.
Des risques de fuites et de piratage informatiques
Au Royaume-Uni, 28 millions d’enregistrements représentant un total de plus de 23 gigaoctets ont été publiés sur internet après l’exploitation d’une faille d’une solution de l’entreprise Suprema, dont les clients sont notamment la Metropolitan Police, des entreprises de défense et des banques dans 83 pays. Outre des noms d’utilisateur et des mots de passe non cryptés, des registres d’accès aux installations, des niveaux de sécurité et des habilitations, les données exposées concernaient aussi les empreintes digitales et les enregistrements de reconnaissance faciale de millions de personnes. En plus du risque de manipulation des systèmes de contrôle d’accès de sites sécurisés, les observateurs ont souligné que le problème le plus grave résidait dans l’accès à des données biométriques qui ne peuvent par nature être modifiées.
Pour ceux qui pensent que l’intelligence artificielle concerne un futur très très lointain, il explique qu’aujourd’hui, en Chine, il existe déjà un système de contrôle social à points, qui a des conséquences directes pour la vie de milliards de citoyens à qui il est interdit de voyager, qui voient leur image affichée en public, qui reçoivent des appels intempestifs parce qu’ils n’auraient pas honoré une dette, pas payé une amende ou pas traversé sur un passage pour piétons. Ce n’est certainement pas le type de système qu’il souhaite pour Bruxelles.
Enfin, une étude européenne sur le sujet a déjà démontré qu’onze pays européen l’utilisent déjà de façon régulière. Les orateurs disposent-ils d’informations quant à une réglementation européenne en la matière ?
L’oratrice se dit choquée d’apprendre que toute caméra de surveillance peut être équipée d’un logiciel de reconnaissance faciale. C’est une chose dont on n’a pas assez conscience aujourd’hui. Elle demande si les caméras privées peuvent également en être équipées.
Other references¶
- [ ] Smita Kheria, Daithi Mac Sithigh, Judith Rauhofer, Burkhard Schafer, «'CCTV Sniffing': Copyright and Data Protection Implications»
- [ ] !Mediengruppe Bitnik, «Surveillance Chess: Hacking into Closed-Circuit Surveillance—Municipal Surveillance as a Subject of Artistic Fieldwork»
- [x]
- [ ] de Vries Patricia & Schinkel William. «Algorithmic anxiety: Masks and camouflage in artistic imaginaries of facial recognition algorithms», Big Data & Society, 6(1), 2019
- [x] Contributors of Wikipedia, «Dazzle Camouflage»
- [ ] Tangible Cloud: artistic practices, counter-narratives to the mainstream vision of digital: cloud computing.
- [ ] Nicolas Malevé, «The exhibitionary complex of machine vision»
- [x] Amrita Khalid, «‘Dazzle’ makeup won’t trick facial recognition. Here’s what experts say will, 2020»
- [x] Hanna Rose Shell, Ni vu, ni connu, Zones Sensibles, 2014
- [x] https://www.wired.com/story/eye-mouth-eye/
- [x] https://technopolice.be/
- [x] «Hypersurveillance policière», dossier d’articles, Médor web, 2021—22
- [ ] Unpleasant Design (2013), by Selena Savić and Gordan Savičić is a website and 2 books on listing designs which are thought to be unpleasant: for example benches for avoid people staying too long or sleeping on it, anti-climb paintings…
- [ ] Evan Selinger and Woodrow Hartzog, «What Happens When Employers Can Read Your Facial Expressions?», in New York Times, Oct. 17, 2019
- [ ] Woodrow Hartzog and Evan Selinger, «Why You Can No Longer Get Lost in the Crowd», in New York Times, April 17, 2019
- [x] Josh Ye, «China drafts rules for using facial recognition technology», Reuters, August 8 2023
Colophon¶
- CONCEPT & RESEARCH
- Stéphanie Vilayphiou
- PROJECT MENTORING
- Claudia Simonelli
- Valentine Fruchart
- ASSOCIATED FABLAB
- Green Fabric, Brussels
- VIDEO PRODUCTION
- Stéphanie Vilayphiou
- MODEL
- Élodie Goldberg
- THANKS
- Valentine Fruchart
- Claudia Simonelli
- Troy Nachtigall
- Ricardo O'Nascimento
- Fabricademy team
- Élodie Goldberg
- Alexandre Leray
- TOOLS
- OpenCV
- Kniterate
- Inkscape
- Gimp
- Krita
- FONTS
- Terminal Grotesque by Raphaël Bastide
- BOOKLET
- Web documentation written with mkdocs.
- Layout in web2print with paged.js.
- LICENSED UNDER CC4R
- https://constantvzw.org/wefts/cc4r.en.html
- PROJECT FILES
- https://gitlab.com/svilayphiou/fabricademy